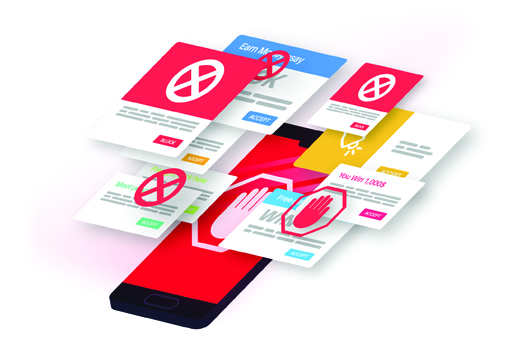
Exploring the Effectiveness of Different Embedding Methods for Toxicity Classification
Abstract
Toxic comments have the potential to negatively impact individuals’ mental health, self-esteem, and overall well-being. Online bullying, hate speech, and harassment often result in emotional distress, anxiety, and even depression among targeted individuals. Furthermore, toxic comments can foster an atmosphere of hostility, division, and polarization within communities, contributing to the erosion of civil discourse and the spread of misinformation. Toxicity classification is an important task in natural language processing (NLP) that involves determining the level of toxicity or offensiveness in a given text. Embedding methods play a crucial role in toxicity classification by transforming textual data into numerical representations that capture semantic and contextual information. This paper explores the efficiency of different embedding approaches for toxicity classification in text, posts or reviews. Particularly, the study compares efficiency of conventional approaches such as CountVectorizer as well as TF-IDF with state-of-the-art approaches including Word2Vec, GloVe, BERT and also GPT3. A large dataset of toxic comments, reviews, or posts is used to evaluate the efficiency of these approaches, employing common metrics like F1-score. The outcomes suggest that GPT3 embedding surpasses all various other approaches accomplishing the greatest F1-score 98.9, followed by Bert and Word2Vec. These results recommend that utilizing pre-trained contextualized embedding can considerably enhance the precision of toxicity classification models.
How to Cite
Al-Daoud, E., Samara, G., Sara, M. R. A., Taqatqa, S., & Kanan, M. (2024). Exploring the Effectiveness of Different Embedding Methods for Toxicity Classification. In Artificial Intelligence and Economic Sustainability in the Era of Industrial Revolution 5.0 (pp. 233-241). Cham: Springer Nature Switzerland.
View at publisher
https://link.springer.com/chapter/10.1007/978-3-031-56586-1_18