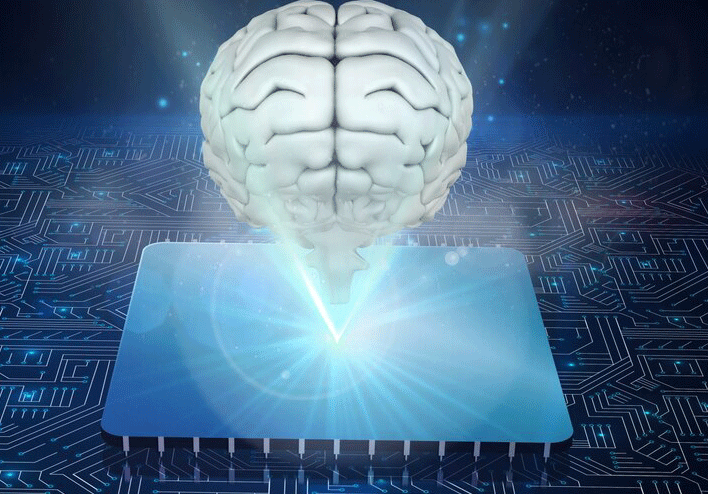
Utilizing Deep Learning Models (RNN, LSTM, CNN-LSTM, and Bi-LSTM) for Arabic Text Classification
Abstract
With the increase in the number of Internet users, the amount of data has increased dramatically. Millions of texts are written daily in most fields, whether cultural, scientific, political, health, or others, through websites, social media, and academic or news institutions. This data has become an essential source for providing additional services. One of these services is the classification of texts into previously defined categories according to the information they contain. Texts in the Arabic language are still considered a great challenge to carry out the task of classifying them, unlike other languages, such as English, French and many other languages. In this research paper, four models of deep learning techniques (RNN, LSTM, CNN-LSTM, and Bi-LSTM) were built to classify texts in the Arabic language, and the models were tested on a data set consisting of news articles and classified based on the content of the article. The highest accuracy result (94.02) was obtained by the (Bi-LSTM) model.
How to cite
Al-Qerem, A., Raja, M., Taqatqa, S., & Sara, M. R. A. (2024). Utilizing Deep Learning Models (RNN, LSTM, CNN-LSTM, and Bi-LSTM) for Arabic Text Classification. In Artificial Intelligence-Augmented Digital Twins: Transforming Industrial Operations for Innovation and Sustainability (pp. 287-301). Cham: Springer Nature Switzerland.
View at publisher
https://link.springer.com/chapter/10.1007/978-3-031-43490-7_22